Enhancing Phishing Detection: A Novel Hybrid Deep Learning Framework for Cybercrime Forensics
Enhancing Phishing Detection: A Novel Hybrid Deep Learning Framework for Cybercrime Forensics
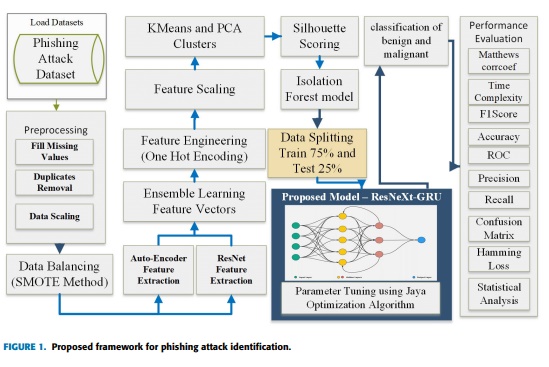
Enhancing Phishing Detection: A Novel Hybrid Deep Learning Framework for Cybercrime Forensics
Boyang Yu1, Fei Tang1, Daji Ergu1, Rui Zeng2, Bo Ma1, and Fangyao Liu1
1College of Electronic and Information, Southwest Minzu University, Chengdu, China
2College of Pharmacy, Southwest Minzu University, Chengdu, China
Corresponding author: Fangyao Liu (FLIU028@163.COM)
Situs web berbahaya menghadirkan ancaman besar terhadap keamanan dan privasi individu yang menggunakannya
internet. Pendekatan tradisional untuk mengidentifikasi situs-situs berbahaya ini sulit untuk diseimbangkan
strategi serangan yang berkembang. Dalam beberapa tahun terakhir, model bahasa telah muncul sebagai solusi potensial
secara efektif mendeteksi dan mengkategorikan situs web berbahaya. Penelitian ini memperkenalkan novel BiDirectional
Model Representasi Encoder dari Transformers (BERT), berdasarkan arsitektur encoder Transformer,
dirancang untuk menangkap karakteristik terkait dari alamat web berbahaya. Selain itu, bahasa berskala besar
model digunakan untuk pelatihan, penilaian kumpulan data, dan analisis interpretabilitas. Hasil evaluasi
menunjukkan efektivitas model bahasa besar dalam mengklasifikasikan situs web berbahaya secara akurat,
mencapai tingkat presisi yang mengesankan sebesar 94,42%. Performa ini melampaui bahasa yang ada
model. Selain itu, analisis interpretabilitas menyoroti proses pengambilan keputusan model,
meningkatkan pemahaman kita tentang hasil klasifikasinya. Kesimpulannya, model BERT yang diusulkan, dibangun
pada arsitektur encoder Transformer, menunjukkan kinerja yang kuat dan kemampuan interpretasi dalam identifikasi
dari situs web berbahaya. Ini menjanjikan sebagai solusi untuk meningkatkan keamanan pengguna jaringan dan melakukan mitigasi risiko yang terkait dengan aktivitas online berbahaya.
source: IEEE
Botnet detection based on network flow summary and deep learning
by Abdurrahman Pektaş, Tankut Acarman
International Journal of Network Management
A botnet is a group of compromised Internet-connected devices controlled remotely by cyber criminals to launch coordinated attacks and to perform various malicious activities. Since botnets continuously adapt themselves to the evolving countermeasures introduced by both network and host-based detection mechanism, the traditional approaches do not provide adequate protection to botnet threat. On the one hand, behavioral analysis of network traffic can play a key role to detect botnets. For instance, behavioral analysis can be applied to observe and discover communication patterns that botnets operate during their life cycle. On the other hand, deep learning has been successfully applied to various classification tasks, and it is also a promising solution for botnet discovery. In this paper, we apply deep neural network to detect botnet by modeling network traffic flow. The performance of the proposed method is evaluated with publicly available large-scale communication traces. The experimental results illustrate that deep learning is an efficient and effective method for identifying botnet traffic with a high true positive rate (attack detection rate) and low false positive alarm rate.
Graph convolutional network for fMRI analysis based on connectivity neighborhood
by Lebo Wang, Kaiming Li, Xiaoping P. Hu
There have been successful applications of deep learning to functional magnetic resonance imaging (fMRI), where fMRI data were mostly considered to be structured grids, and spatial features from Euclidean neighbors were usually extracted by the convolutional neural networks (CNNs) in the computer vision field. Recently, CNN has been extended to graph data and demonstrated superior performance. Here, we define graphs based on functional connectivity and present a connectivity-based graph convolutional network (cGCN) architecture for fMRI analysis. Such an approach allows us to extract spatial features from connectomic neighborhoods rather than from Euclidean ones, consistent with the functional organization of the brain. To evaluate the performance of cGCN, we applied it to two scenarios with resting-state fMRI data. One is individual identification of healthy participants and the other is classification of autistic patients from normal controls. Our results indicate that cGCN can effectively capture functional connectivity features in fMRI analysis for relevant applications.
Convolutional neural networks: an overview and application in radiology
by R Yamashita, M Nishio, RKG Do, K Togashi
Convolutional neural network (CNN), a class of artificial neural networks that has become dominant in various computer vision tasks, is attracting interest across a variety of domains, including radiology. CNN is designed to automatically and adaptively learn spatial hierarchies of features through backpropagation by using multiple building blocks, such as convolution layers, pooling layers, and fully connected layers. This review article offers a perspective on the basic concepts of CNN and its application to various radiological tasks, and discusses its challenges and future directions in the field of radiology. Two challenges in applying CNN to radiological tasks, small dataset and overfitting, will also be covered in this article, as well as techniques to minimize them. Being familiar with the concepts and advantages, as well as limitations, of CNN is essential to leverage its potential in diagnostic radiology, with the goal of augmenting the performance of radiologists and improving patient care.
• Convolutional neural network is a class of deep learning methods which has become dominant in various computer vision tasks and is attracting interest across a variety of domains, including radiology.
• Convolutional neural network is composed of multiple building blocks, such as convolution layers, pooling layers, and fully connected layers, and is designed to automatically and adaptively learn spatial hierarchies of features through a backpropagation algorithm.
• Familiarity with the concepts and advantages, as well as limitations, of convolutional neural network is essential to leverage its potential to improve radiologist performance and, eventually, patient care.
by Zonghan Wu; Shirui Pan; Fengwen Chen; Guodong Long; Chengqi Zhang
Kampus 4
Jalan Ahmad Yani (Ringroad Selatan) Tamanan, Banguntapan, Bantul, Yogyakarta 55166
Telepon : (0274) 563515, 511830, 379418, 371120
Faximille : 0274-564604
Email : prodi[at]tif.uad.ac.id
Daftar di UAD dan kembangkan potensimu dengan banyak program yang bisa dipilih untuk calon mahasiswa
Informasi PMB
Universitas Ahmad Dahlan
Telp. (0274) 563515
Hotline PMB
S1 – 0853-8500-1960
S2 – 0878-3827-1960
© 2021-2023 Program Studi Informatika | Home |